“>80% of brands globally are expected to adopt technologies to analyze customer sentiment on social media by 2023 - Bain & Company.”
Influencer marketing traditionally has relied heavily on broad metrics like follower count and engagement rates. Brands have used audience demographics and conversion tracking to find relevant influencers and evaluate their ROI.
However, using quantitative metrics only has its limitations. Some of them are:
- Lack of Context: Metrics alone may need to provide more context or insights into the overall performance of an influencer campaign. Factors like campaign objectives and brand fit also play significant roles in evaluating success, which quantitative metrics cannot adequately capture.
- Vanity Metrics: Metrics such as follower count, likes, and comments can be easily inflated. They do not always reflect the actual engagement or impact of an influencer's content.
- Influencer Fraud: The prevalence of fake followers, engagement pods, and bot activity challenges the accuracy of metrics. Some influencers may use deceptive practices to artificially boost their metrics, making it difficult to rely solely on those metrics for campaign evaluation.
- Evolving Algorithms: Social media platforms regularly update their algorithms, which can impact the visibility and reach of influencer content. Metrics can fluctuate due to algorithm changes, making it challenging to accurately establish consistent benchmarks or measure long-term performance.
With such limitations, brands often need help attributing their campaigns' success or failure to the influencers. To get a more in-depth understanding of things, marketers also need to use qualitative tools.
One such tool is - Sentiment Analysis.
What is Sentiment Analysis?
Sentiment analysis is an artificial intelligence (AI)-based capability that uses machine learning to recognize sentiments and assess the emotional content of texts and images. It is a layer of understanding applied to the rest of your social media metrics that put the acquired data into context.
With sentiment analysis, marketers try to understand people’s thoughts and feelings based on what they write, photograph, or say about something (e.g., products, people, companies, and organizations). Social media sentiment analysis provides context for your customers’ conversations around the social space.
Can your product evoke a comforting and pleasant sensation in customers? Are you fulfilling their expectations in terms of service quality? Sentiment analysis can provide answers to these inquiries and more.
However, despite their advantages, sentiment analysis tools come with certain limitations:
What are the limitations of current Sentiment Analysis Tools?
Despite their utility for qualitative measurements, most sentiment analysis tools show apparent limitations.
Current sentiment analysis tools rely on the volume of sentiment-related terms. However, this doesn’t always tell the full story. Let’s see this Netflix’s Facebook page, for example:

While fans express their admiration for the programming, they also use terms like "ugly," "cry," and "depressed" in their feedback. Without proper context, encountering such terms in mentions could be concerning.
Sentiment analysis can be perplexed by sarcasm as well. For instance, when someone tweets, "I love it when I lose my luggage after a nine-hour flight," their statement indicates dissatisfaction despite using sarcastic language.
Although sentiment analysis can provide insights into your brand's public emotions, such a scenario shows why current tools cannot produce accurate results.
Another issue with current sentiment analysis tools is that these tools provide broad, overall sentiment for a particular brand or product. Social media listening tools like Sprinklr and Meltwater typically segregate the sentiments into positive, negative, or neutral.

While this helps understand how audiences perceive content and brands, it doesn't address meaningful interactions. For example, consider this sponsored post by Mamaearth with an influencer:

A simple scroll through of the comments reveals that most are men showering hearts, followed by some relevant comments that enquire about the quality, side effects, and price.
Therefore, the simple number of comments, engagements, or even brand mentions doesn’t produce the true picture of audience sentiments. It is now apparent that current sentiment analytics need to be backed with intelligent context.
- Do not give importance to the context
- Cannot point out meaningful interactions
- Are unable to gauge purchase intent
Phyllo’s solution: Engagement Quality Analysis
The sentiment of a comment can be judged fairly, even without any context. However, the relevance of a comment is highly contextual ( as relevancy is dependent on the post).
Marketers and developers of the influencer marketing community want to analyze both - the sentiment and relevancy of the comments for a particular post in one place.
Phyllo has trained its tool with a Large language model (LLM) and a large dataset of words with positive/negative connotations to detect “positive” and “negative” sentiments and score the comment accordingly. It’s a complete sentiment analysis solution that provides traditional overall sentiment scores and an AI-backed relevance score.

Phyllo’s Engagement Quality Analysis assigns a relevance score to the total comments in a post and surfaces relevant comments to the marketer. It further segregates positive engagements into:
- Highly relevant and important: Most relevant and offer substantial insight. Examples: testimonials, strong purchase intent, expert advice
- Relevant and useful: Relevant and contain important perspectives. Examples: Basic product feedback, tagging other users, constructive criticism
- Moderately relevant: Related to the brand but does not offer significant value. Examples: General statement or fact about the brand
- Slightly relevant: Off-topic and weak connection to the brand. Examples: Unrelated discussions, emojis without supporting text
- Completely irrelevant: Unrelated to the brand/product. Examples: Spam, personal conversations.
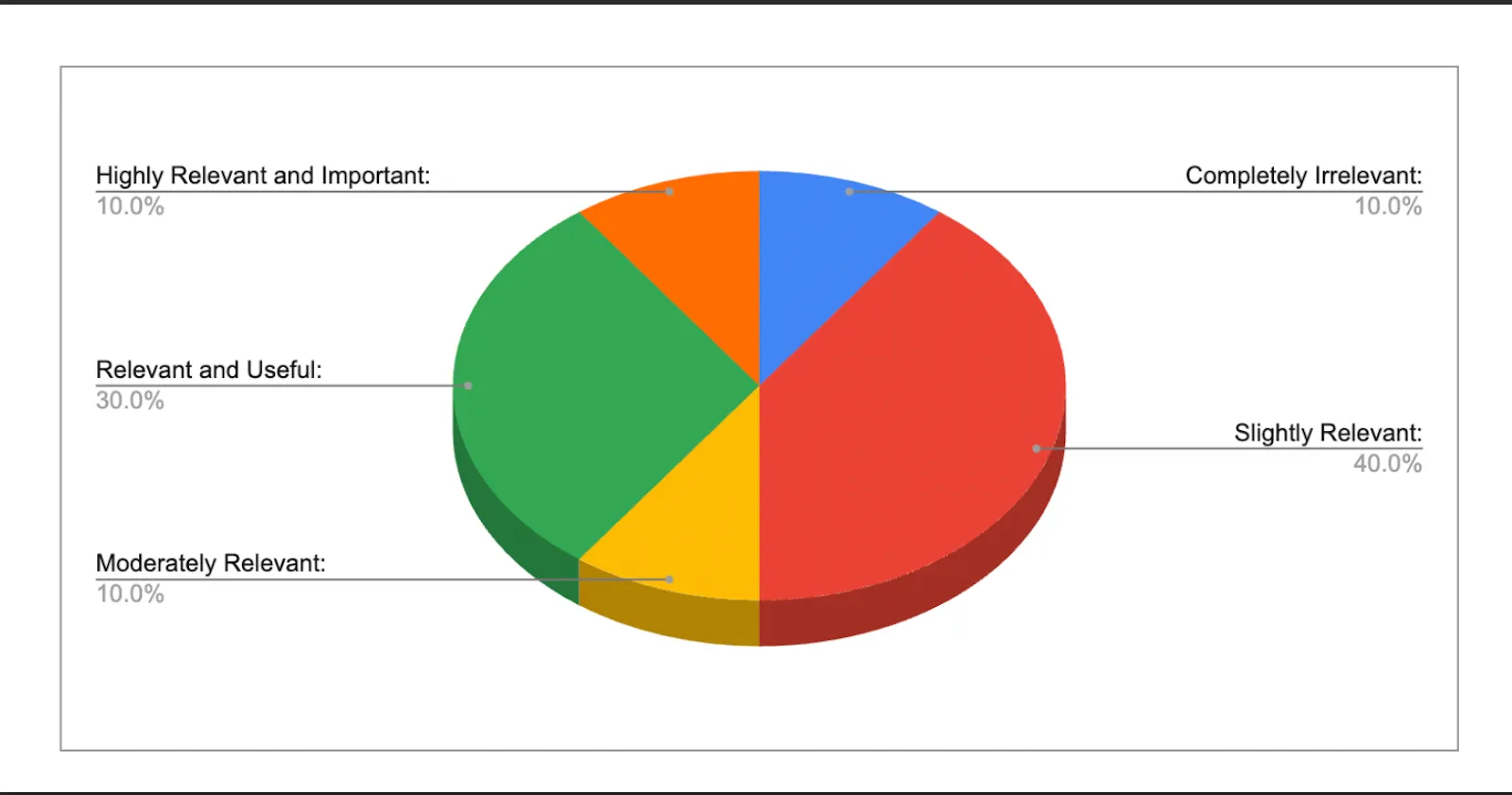
Influencer and brand marketing managers can use our real-time opinion analysis tool to:
- Identify influencers who are a brand fit and drive the best ROI
- Save 100s of hours over manually analyzing engagement on content
- Find engaged audience profiles and target similar ones using ads
- Stay updated with the pulse of campaigns and communicate faster with customers
- Collect product reviews and testimonials and identify customer champions
- Understand the product mix that creates the maximum impact
Phyllo’s Engagement Quality Analysis is available as an API or as part of Phyllo’s dashboard.
To know more about Phyllo’s AI sentiment analysis tool, contact us now!
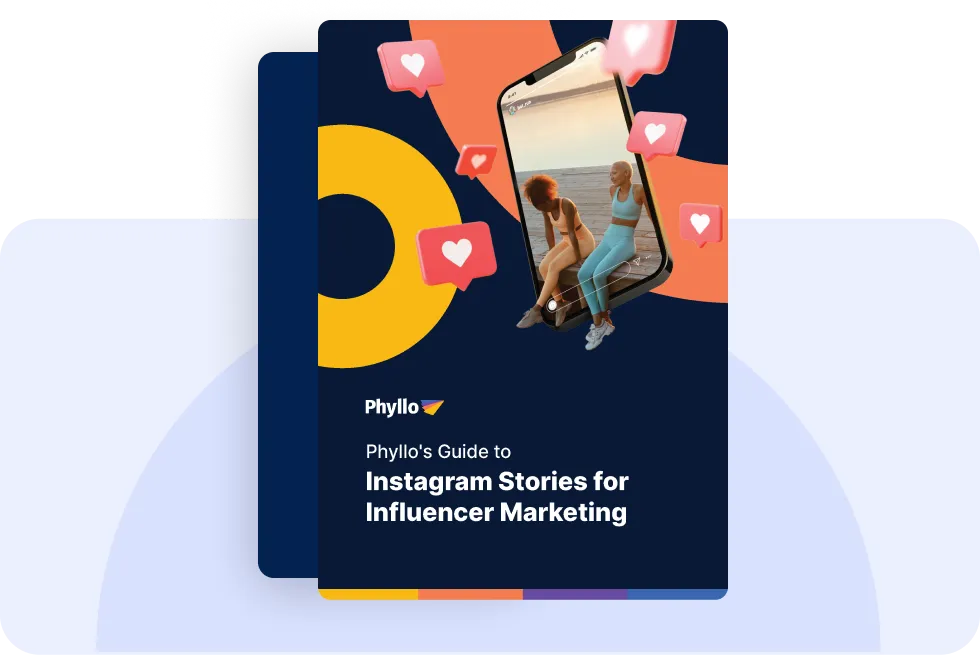